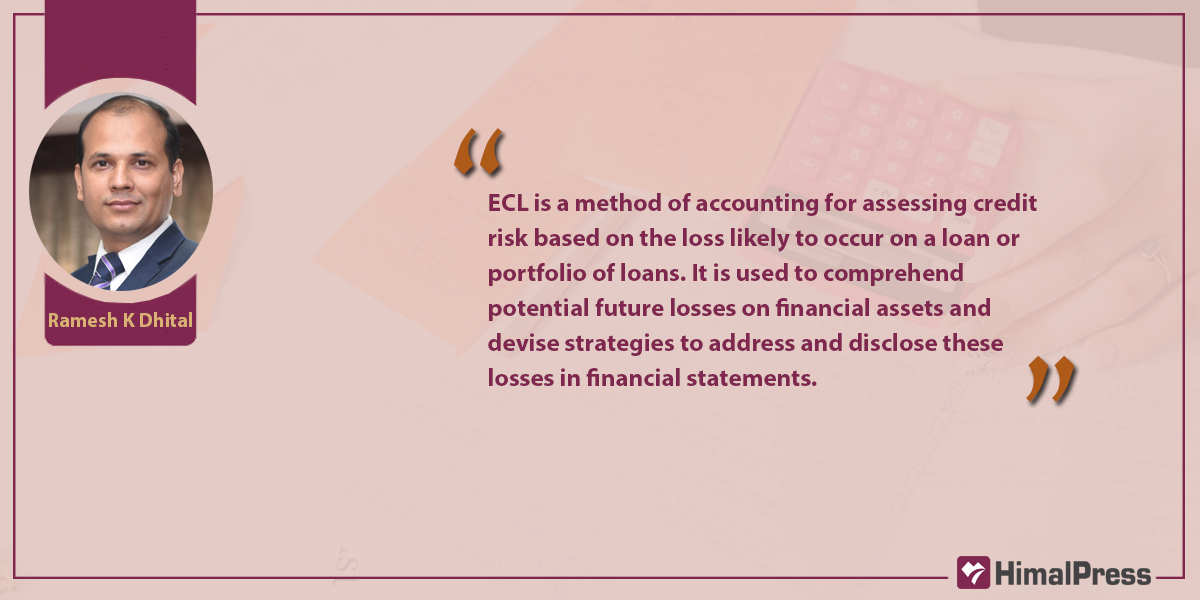
Nepal Rastra Bank (NRB) recently issued directives to banks and financial institutions (BFIs) instructing them to update the central bank on their preparedness status regarding the development of the Expected Credit Loss (ECL) Model, the enhancement of human resource capacity, and other relevant preparatory tasks associated with ECL Implementation.
The Institute of Chartered Accountants of Nepal (ICAN), the regulatory body for accounting, has provided a carve-out with an alternative treatment in Nepal Financial Reporting Standards (NFRS) 9 Para 5.5 (impairment) until the fiscal year 2023/24.
Accordingly, BFIs are required to align their financial statements, including quarterly reports, with NFRS 9, effective from the first quarter of the fiscal year 2024/25. The implementation of the new accounting requirement under NFRS 9 will necessitate BFIs to establish provisions for expected credit losses which will require the current banking systems to develop and implement new systems for evaluating all categories of financial assets in accordance with NFRS 9.
NFRS 9 provides a forward-looking approach, outlining the requirements for provisioning based on the expectation of credit losses (ECL) even at the initial recognition of financial assets such as loans and investments.
What is ECL?
ECL is a method of accounting for assessing credit risk based on the loss likely to occur on a loan or portfolio of loans. It is used to comprehend potential future losses on financial assets and devise strategies to address and disclose these losses in financial statements.
Through ECL, banks can gauge the likelihood of default for each loan. By multiplying this probability by the expected loss given default, the bank determines the anticipated percentage loss in the event of borrower default. The resulting value, when multiplied by the probable exposure at default, is the expected loss for each loan, and the sum of these values is the expected loss for the entire portfolio.
The incurred loss approach is often criticized for overlooking the early warning signals about deteriorating asset quality and leading to a delayed identification of credit losses. The ECL model prompts banks to look for early warning signals which can help in the identification of potential credit losses and facilitate pre-emptive actions.
The ECL model, proposed by accounting regulators, encourages early recognition of stress, in contrast to the traditional approach where provisions are made after losses are incurred. This shift aims to enhance the resilience of the banking system. As banks transition from the incurred-loss approach to the ECL model, they will be allowed to design their credit loss models with this requirement.
The adoption of the ECL model will require banks to classify loans into three stages based on the asset’s overdue position. Banks will have to compute the probability of default (PD), loss given default (LGD), and exposure at default (EAD) at the loan level, based on historical data of borrowers in various portfolios.
ECL formula = EAD x PD x LGD x Discount Factor
The aim is to enable BFIs to take early action to mitigate potential credit losses, ultimately reducing the impact of credit risk on their financial performance. The responsibility for credit risk practices and the adequacy of ECL frameworks lies with the board and senior management of these institutions.
Embedding Macro-Economic Data, Statistics
The historical Probability of Default (PD) derived from internal historical data of banks has to be calibrated with forward-looking macroeconomic factors to determine the PD term structure. Lognormal graphs are employed to extrapolate this data, with Economic Factor Adjustment (EFA) playing a pivotal role in ECL implementation. EFA, a component of the ECL calculation, adjusts provisions based on anticipated future economic conditions, encompassing factors like GDP growth rate, unemployment rate, inflation, interest rates, exchange rates, regulatory impact, and government policies. Weighting for specific variables is determined through mock test calculations and industry data averaging facilitated by central banks.
Preparation and Considerations
Implementing the ECL framework presents some challenges for banks such as insufficient volume of historical default data required to build robust PD models if segment-wise risk profiles are not maintained effectively. Banks need to have robust and flexible systems capable of conducting intricate calculations promptly using extensive data from multiple sources. Initiating a data cleansing project may be necessary to ensure deal-wise uniform data for conclusive computations. Pricing models for banks must align with expected loss models to account for such costs at the acquisition stage.
The unavailability of human resources and relevant accounting and statistical analytical skills may hinder implementation as the team needs to understand, build, and manage ECL models even manually initially. Challenges may also arise in building the right model, complying with uncertain regulatory requirements, streamlining systems and processes, and training employees. The implementation of ECL could result in higher provisioning costs for banks that may impact their profitability in the short term. Changes in assumptions and parameters may significantly alter ECL results, leading to high volatility in financial results that may impact growth and risk mitigation plans for banks.
Despite these challenges, the introduction of ECL guidelines is a positive step in the right direction. Banks must build systems to adopt a more proactive approach to credit risk management. The adoption of ECL would lead to banks adopting more data-driven analytical models for credit risk management. While the migration to ECL poses implementation challenges, banks must take proactive measures to ensure successful adoption and compliance with regulatory requirements.
Regulatory Approval and Implementation of ECL Reporting
Regulatory acceptance is an important consideration in ECL reporting from an implementation perspective. Research indicates that in economies like Sri Lanka, the Maldives, and Bhutan, where data retention may not meet the standards required for ECL, regulators have followed minimum observation periods of 3-5 years before aligning prudential regulations with ECL requirements outlined in NFRS 9.
Such alignment could result in significant modifications to the preliminary models developed by BFIs. Hasty system implementation could impede the ability of the users to understand credit modeling principles and the interpretation of corresponding outcomes. Common challenges include management struggling to interpret ECL system outputs and reconcile them with the core business.
Model maturity will define the stability of the model under various economic conditions. Preliminary models can be engaged in a parallel run, allowing for back-testing and recalibration. However, such validation exercise within system parameters may lead to a costly exercise with numerous change requests.
Hence, manual computation in the initial years presents an opportunity to gain confidence in the numbers, analyze the factors of sensitivity, and utilize discounting factors based on the estimated life of assets and their impact on result interpretation.
Moving forward
The ECL implementation can be started by establishing a project steering committee comprising members from the Finance, Credit Risk, Internal Audit, and Information Technology departments, along with industry experts. Drawing insights from reference documents provided by accounting regulators and central banks in neighboring countries, the committee can formulate an implementation plan. The committee may also propose and discuss a Governance Structure for ECL implementation, outlining the roles of various entities such as the Board and Audit Committee, Finance Committee, Credit Risk Management Team, IT Team, and Internal Audit.
This committee can engage in detailed research and internal discussions on the Classification of Financial Assets, Contractual Cash Flow Assessment, Business Model Assessment, Expected Credit Loss Model (ECL), Financial Asset Segmentation, Staging Criteria, Development of ECL Model and its key functions, assumptions/estimates to be utilized in ECL model development and consider the annual model validation requirements conducted by an independent third party.
(CA Dhital is a member of the Institute of Chartered Accountants of Nepal.)